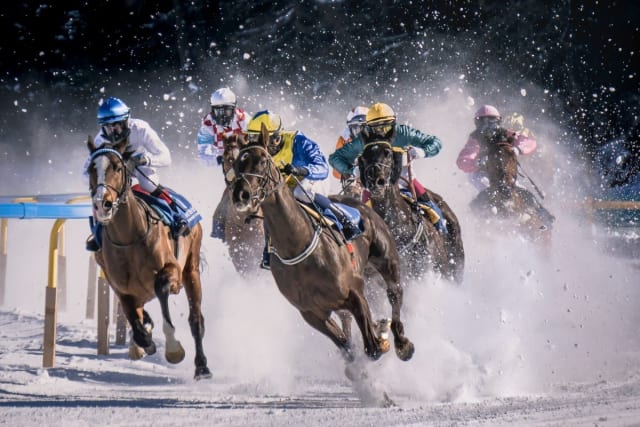
The Anatomy of an Arbitrage Betting Bot
- Alex McConnell, Cybersecurity Content Specialist
5 minutes read
In gaming and betting, it is said that the house always wins. However, some bettors are constantly looking for loopholes to guarantee a profit no matter the outcome of their bets. They have even developed sophisticated software tools to help with a controversial tactic called arbitrage betting, which costs the industry millions each year.
In this blog we’ll look at how arb betting works, the arb bot tools being used to facilitate it, and how bookmakers are fighting back against this troublesome practice.
What is arbitrage betting?
Arbitrage betting, or arb betting, is a tactic used to guarantee a profit when making a series of opposing bets on the same event.
First, the arb bettor places a bet on an event with a bookmaker or betting site, before “laying” that bet on the same event using a betting exchange – in other words, betting on the opposite outcome. Essentially the arb bettor is selling the bet for more than they bought it for, so that no matter the outcome of the bet, they will turn a profit.
For example, in a World Cup football match with no possibility of a draw between England and Sweden:
Bookmaker A has Sweden at 8/15 (naturally, Sweden are the best!) and England at 12/7, and bookmaker B has Sweden at 2/5 and England at 9/4.
You bet £100 on Sweden with bookmaker A and £47.18 on England with bookmaker B.
If Sweden wins, you get a return of £100 *(1+ 8/15) = £153.3, if England wins you get a return of £47.18 *(1+ 9/4) = £153.3. From your bet of £147.18, so you are guaranteed a profit of £153.3 – £147.18 = £6.12.
Arb-betting is not illegal and is even encouraged by some bookmakers. However, most big-name bookmakers consider arbers to be a liability, and as such, it is an industry-wide problem.
Why are bots essential for arb betting to be effective?
Bets that will yield a profit in this way exploit odds imbalances, which are often hard to come by and exist mainly in smaller or niche sports. Suitable events that generate opportunities for a profitable arbitrage bet are also time consuming to find, and do not yield a big profit individually.
Arb bettors can make the practice worthwhile by using tools, like arb bots, that automate the process of finding these opportunities, whilst calculating the profit they are guaranteed to return.
Netacea’s threat research team has been supporting our clients in the gaming and betting industry by researching some of the most popular arb betting automation tools for this purpose.
How do arb betting bots work?
Bot attacks are often made up of several distinct actions or stages (for more information see the BLADE™ – or Business Logic Attack Definition – framework). Our research revealed that, at their core, arb betting bots are facilitated by scraper bots.
These arb bots “scrape” (or extract) the content from multiple bookmaker websites and betting exchanges, specifically the up-to-the-minute odds for available bets, and feed this information into an algorithm to determine where arb bets can be made for guaranteed profit.
Some arb betting bots are even capable of automating the entire process of placing and laying the bets, using similar functionality for this stage as a retail or ticket scalper bot.
In one such arb betting tool, the user is presented with a list of arbitrage betting opportunities. Each is given a profitability rating based on the current odds on a bookmaker website versus the same lay bet on an exchange. This rating is based on the guaranteed payout for a bet of £100 – for example, if the rating is 120, the user is guaranteed a return of at least £120 minus their £100 stake, no matter the outcome.
The best profitability rating we saw whilst investigating one such tool was 134.44, promising a profit of £34.44 on a £100 bet. These tools are thus important for arb bettors to maximize their efficiency.
However, odds can change rapidly, and most opportunities are only available for a very short period, making arb betting a time-sensitive operation. As a result, the scraper bots feeding data into arb betting tools often attack their targets aggressively to ensure the information is always accurate.
How much are arb betting bots costing bookmakers?
Bookmakers want to stop arbitrage bettors because these people take advantage of loopholes to always make a profit. Arb bettors are not taking on the most profitable bets for the bookmakers, so serving them costs the bookmakers money in the long run.
Aggressive scraping activity by sports betting bots also adds unwanted costs to hosting infrastructures, as the bookmakers are forced to serve this traffic despite it not coming from legitimate customers. The cost of doing this can be significant, reaching millions of dollars per year, as scrapers account for most of the traffic making requests on a bookmaker’s site or API at peak times.
How to prevent arb-betting with Netacea
Netacea recently helped a leading bookmaker with some problems that arb-betting had been causing their own business, as arb-betting has the potential to cost bookmakers thousands in customer payouts. By monitoring all sessions on the bookmaker’s website, we were able to build a ‘cluster’ of sessions to identify when and where arb-betting bots were being deployed. We quickly identified the worst of scraper bots - carefully picking them out amongst the good bots crawling the site – and were able to implement systems that block these bots automatically.
Since we began blocking the scrapers, our best estimate is that arb-bot activity has reduced by approximately 85%, even creating faster response times and lowering infrastructure costs as a result.
Subscribe and stay updated
Insightful articles, data-driven research, and more cyber security focussed content to your inbox every week.
By registering, you confirm that you agree to Netacea's privacy policy.